Categories
Archives
- July 2024
- June 2024
- May 2024
- March 2024
- January 2024
- December 2023
- October 2023
- September 2023
- August 2023
- July 2023
- May 2023
- April 2023
- January 2023
- December 2022
- October 2022
- September 2022
- July 2022
- June 2022
- April 2022
- March 2022
- December 2021
- November 2021
- September 2021
- July 2021
- May 2021
- March 2021
- January 2021
Extract, Transform, and Load (ETL)
ETL pipelines process data in a linear fashion with a different step for each phase. They first collect data from different sources, transform the data to remove dirty data and conform to business rules, and load the processed data into a destination data store. This approach has been used in business intelligence (BI) solutions for years and has a wide array of established best practices. Each of the three phases requires an equal amount of attention when being designed. If properly designed and developed, ETL pipelines can process multiple sources of data in parallel to save time. For example, while data from one source is being extracted, a transformation activity could be working on data that has already been received, and a loading process can begin working on writing the transformed data to a destination data store such as a data warehouse. Figure 1.11 illustrates common Azure technologies used in each phase of an ETL workflow.
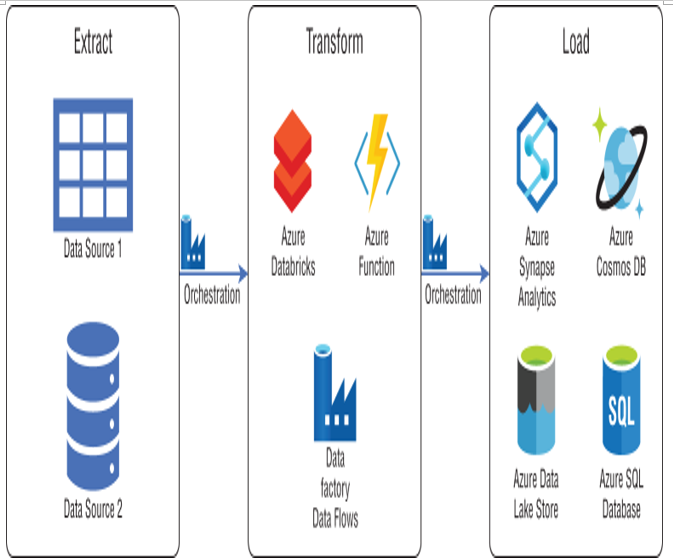
FIGURE 1.11 ETL workflow
In this example, data is extracted from its source systems and transformed by one or more compute engines such as Azure Databricks, Azure Functions, or Azure Data Factory mapping data flows. After the necessary transformations are completed, the data is loaded into a destination data store such as Azure Synapse Analytics, Azure Cosmos DB, ADLS, or Azure SQL Database to power different types of applications. ADF automates this workflow and controls when each step is executed. Keep in mind that this is a rudimentary example and a typical ETL pipeline may include several staging layers and transformation activities as data is prepared. The following sections describe each phase and how each activity is managed in an ETL workflow.
Leave a Reply